The information age has opened the floodgates for data like never before. With this deluge comes a burgeoning requirement to extract meaningful insight from it. In its various forms, interaction analytics has turned out to be a strong tool in deciphering this deluge of data that is fast changing industries and decision-making processes.
We will cover in detail voice, audio, speech, text, video, and conversational analytics with regard to their differences, uses, evolution over time, and what the future holds. It will further contain some of the emerging trends like augmented analytics and generative AI to give an all-rounded view of fast-changing areas.
The Analytics Landscape: Understanding
While these terms are used interchangeably, voice, audio, speech, text, video, and conversational analytics, there are very distinct characteristics and focus areas.
Voice Analytics
Voice analytics is applied to the acoustic properties of speech, including pitch, tone, and volume. It can be used in inferring emotional states, identifying speakers, and detecting stress levels.
Examples and Case Studies
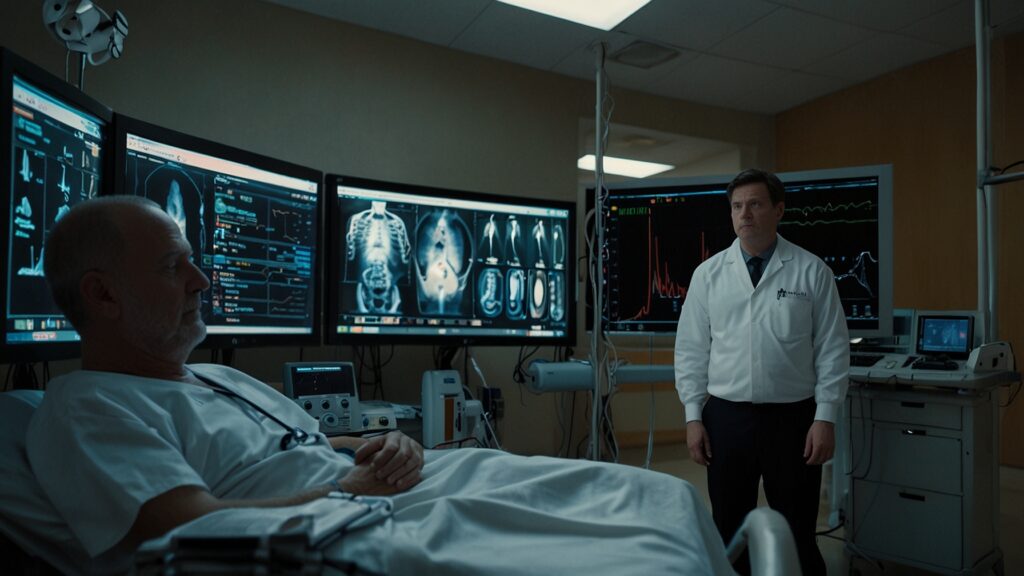
Healthcare: The application of voice analytics has been tried in monitoring patients with mental health conditions. Researchers have analyzed speech patterns for tell-tale signs of depression.
Customer Service: Voice analytics helps companies enhance customer service experiences by analyzing the emotional pitch of callers and modulating responses in line to achieve better customer satisfaction.
Trends
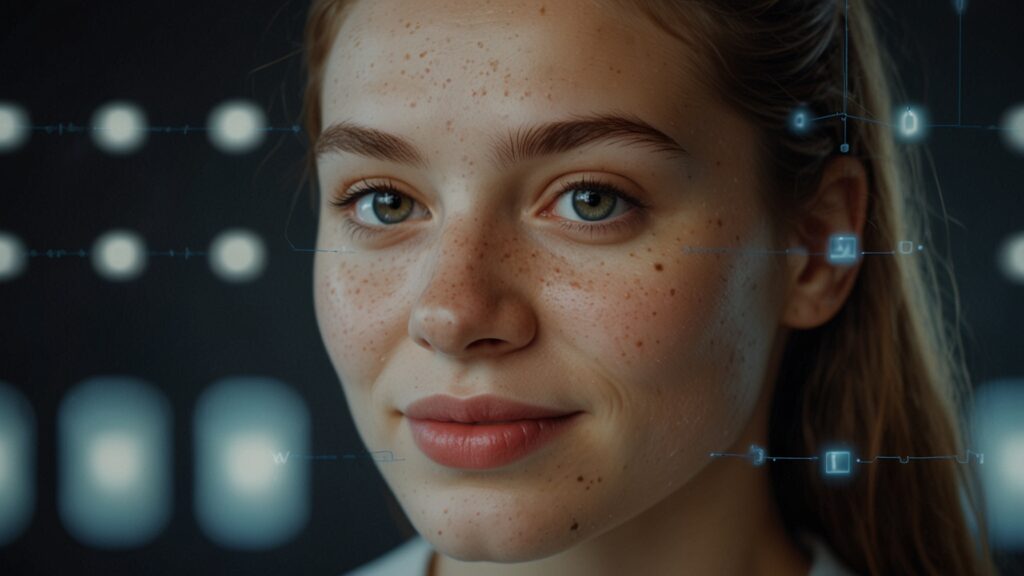
Emotion AI: Improvements in Emotion AI allow for the detection of subtle emotions that help systems recognize a more significant number of emotional states and tone shifts, leading to more nuanced and empathetic human-computer interactions.
Voice Cloning: Very realistic synthetic voices generated with the help of AI-powered voice cloning technology open a lot of opportunities but also a lot of ethical concerns, raising questions about identity, privacy, and potential misuse.
Audio Analytics
Audio analytics is a broad area including the analysis of sound; music, environment noises, and speech. It involves sound classification, noise reduction, and pattern recognition.
Examples and Case Studies
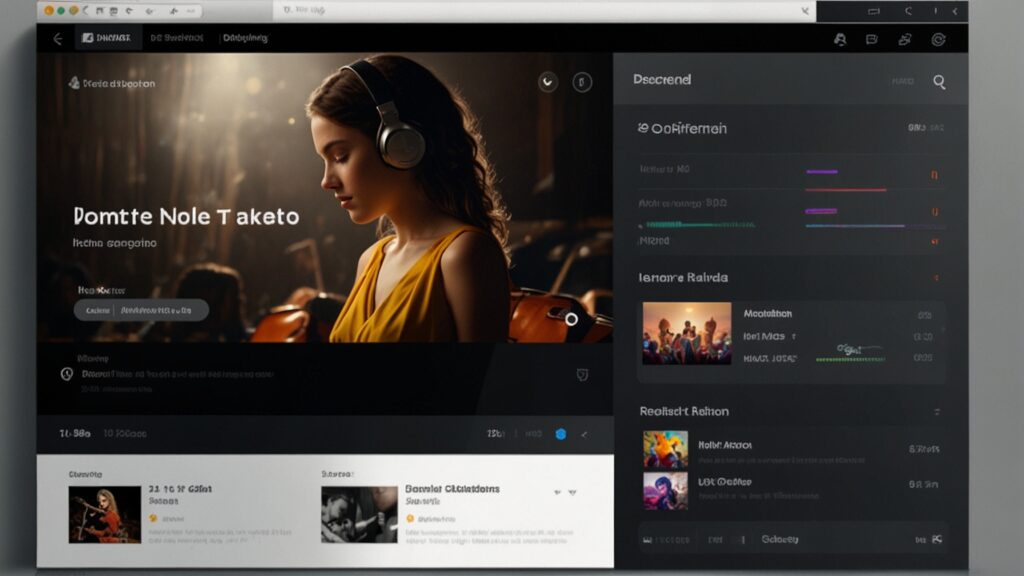
Manufacturing: Businesses apply audio analytics to understand the sounds of machines better in order to anticipate maintenance and avoid failures. This saves them many costly breakdowns.
Entertainment: Streaming services such as Spotify utilize audio analytics in enhancing music recommendation algorithms by analyzing listening patterns and audio characteristics.
New Trends
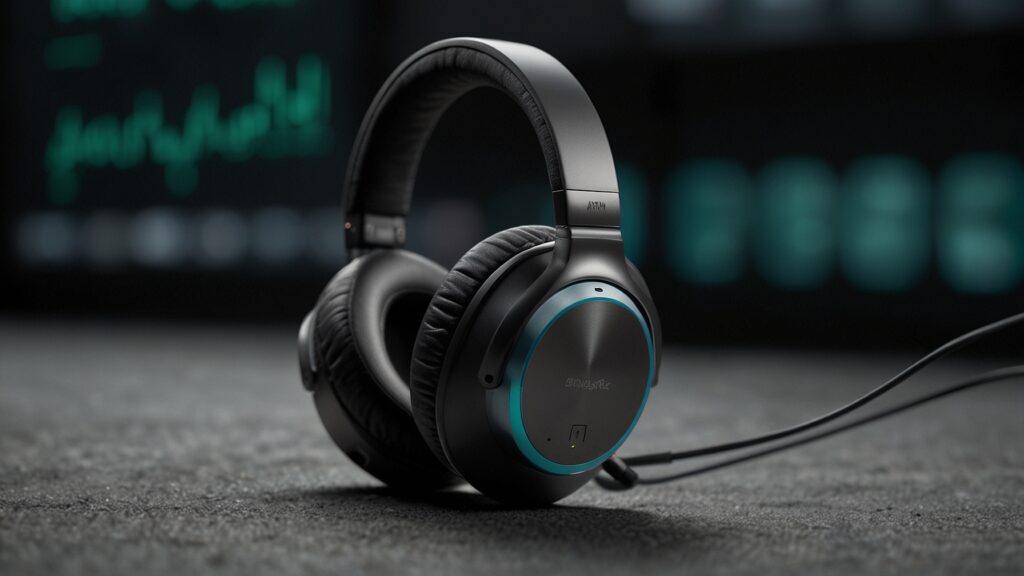
Real-time Noise Cancellation: Creation of sophisticated algorithms for real-time noise cancellation in consumer headphones and industrial environments, leading to improved focus, productivity, and overall audio experience.
Soundscapes: Audio analytics for generating and analyzing immersive soundscapes in virtual and augmented reality environments is getting more and more important, enhancing user experiences and creating new opportunities for storytelling and emotional engagement.
Speech Analytics
Speech analytics focuses on the linguistic content of spoken language. It involves speech-to-text, after which it analyzes the transcribed data for keywords, topics, sentiment, and other textual attributes.
Examples and Case Studies
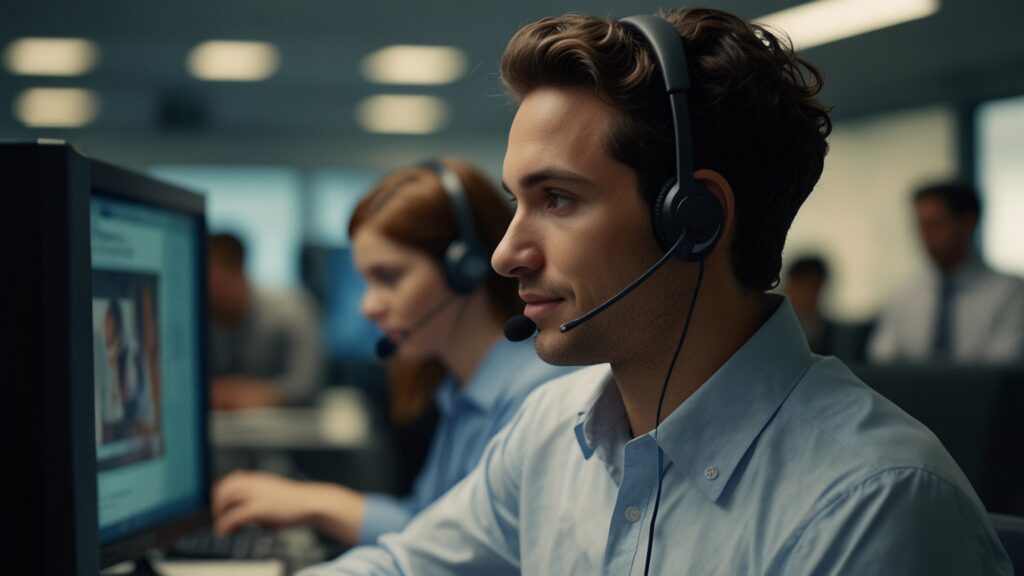
Call Centers: Companies analyze customer service interactions using speech analytics to identify trends, optimize agent performance, and drive customer satisfaction, ultimately leading to improved customer experiences and increased loyalty.
Legal Domain: Speech analytics is applied to transcribe and analyze courtroom proceedings to assist in the legal documentation process and case management, ensuring accuracy, efficiency, and accessibility to legal information.
Emerging Trends
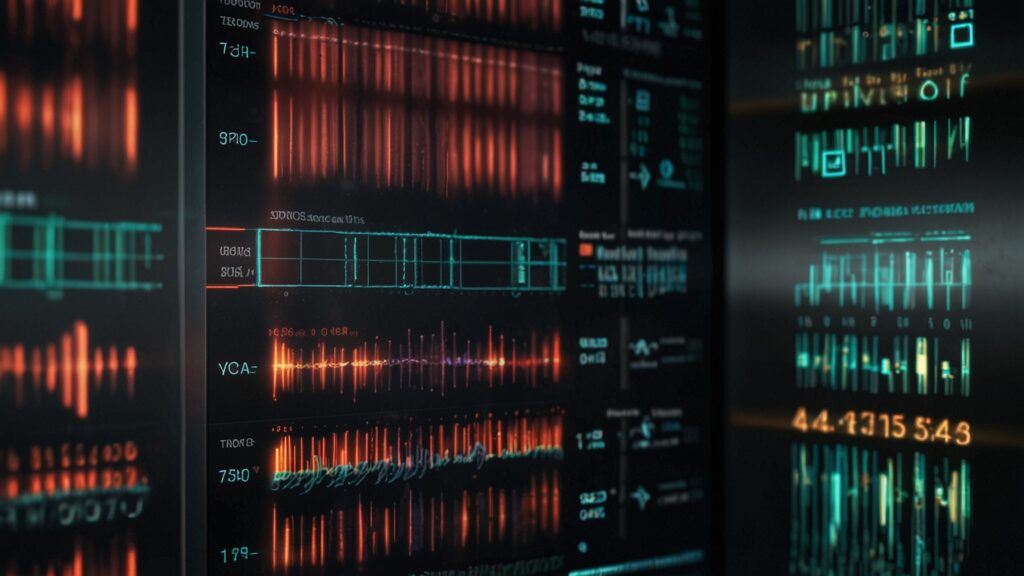
Speech-to-Text Enhancements: Enhancement in NLP technologies is making speech-to-text conversion more accurate, even in noisy environments as well as with strong accents, expanding accessibility and utility across various applications.
Voice Biometrics: The merger of speech analytics with biometric systems is increasing security through the usage of voice prints to authenticate a person, providing a robust and convenient alternative to traditional authentication methods.
Text Analytics
Text analytics is the process of taking raw textual data and then deriving meaningful insights and patterns from it. This is made possible with techniques such as natural language processing for understanding and deciphering text.
Examples and Case Studies
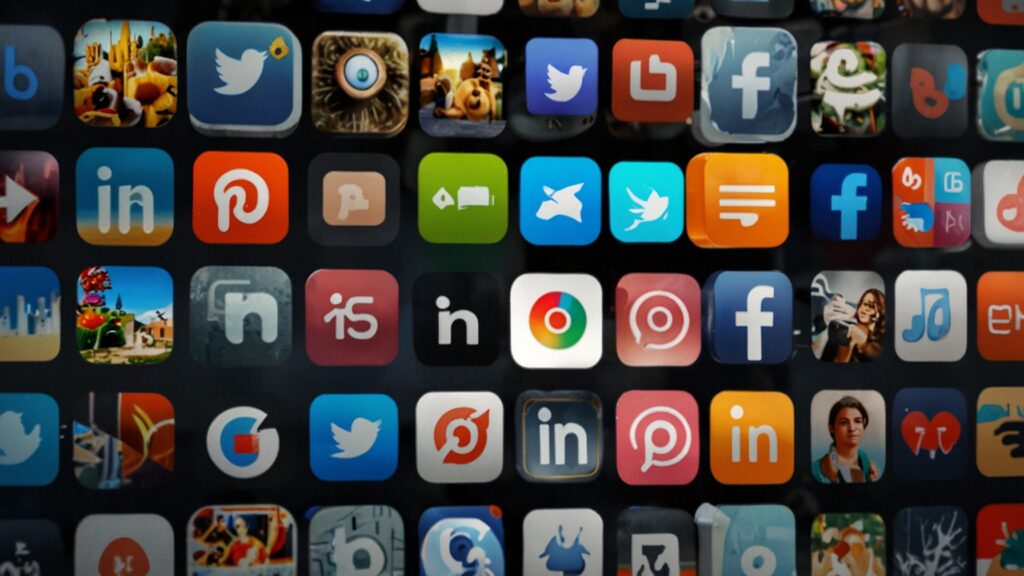
Social Media Monitoring: Social media companies analyze social media posts for brand sentiment and identifying emerging trends to help companies tailor their marketing strategies, enhancing brand reputation and customer engagement.
Fraud Detection: Text analytics is applied in financial institutions to check for suspicious trends in transaction descriptions, which might point to fraudulent activities, safeguarding financial assets and protecting customers from financial loss.
Trends
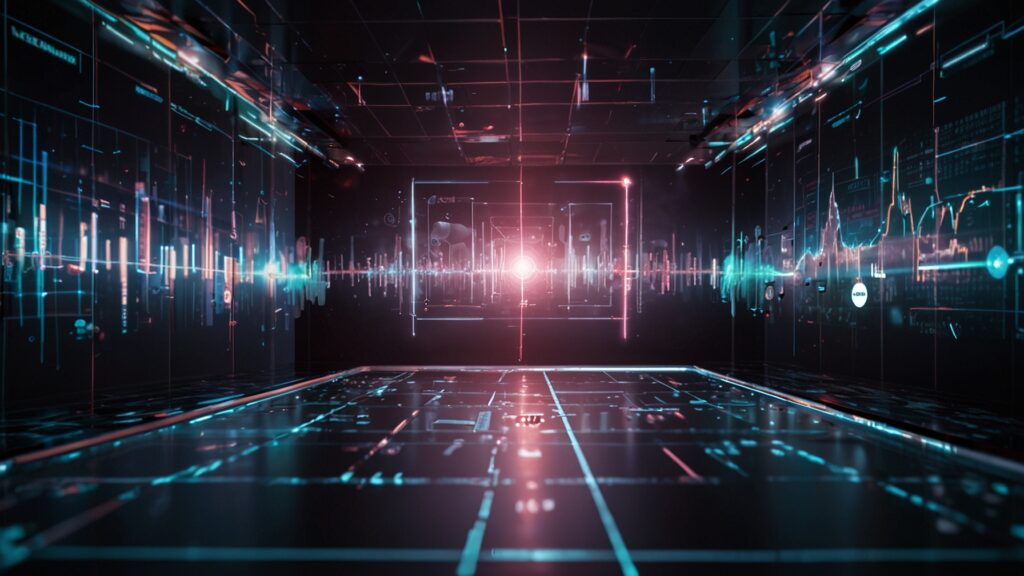
Augmented Analytics: Using AI for automation of user management of data mining and analysis, augmented analytics will enable users to find insights more rapidly and intuitively, democratizing data and empowering businesses to make data-driven decisions faster.
Generative AI: Models like GPT-4 will help in the creation of text creation and advanced understanding, developing very complex content and interaction applications, revolutionizing fields from content creation to customer service.
Video Analytics
Video analytics extracts information from video content to include object detection, facial recognition, motion analysis, and sentiment analysis.
Examples and Case Studies
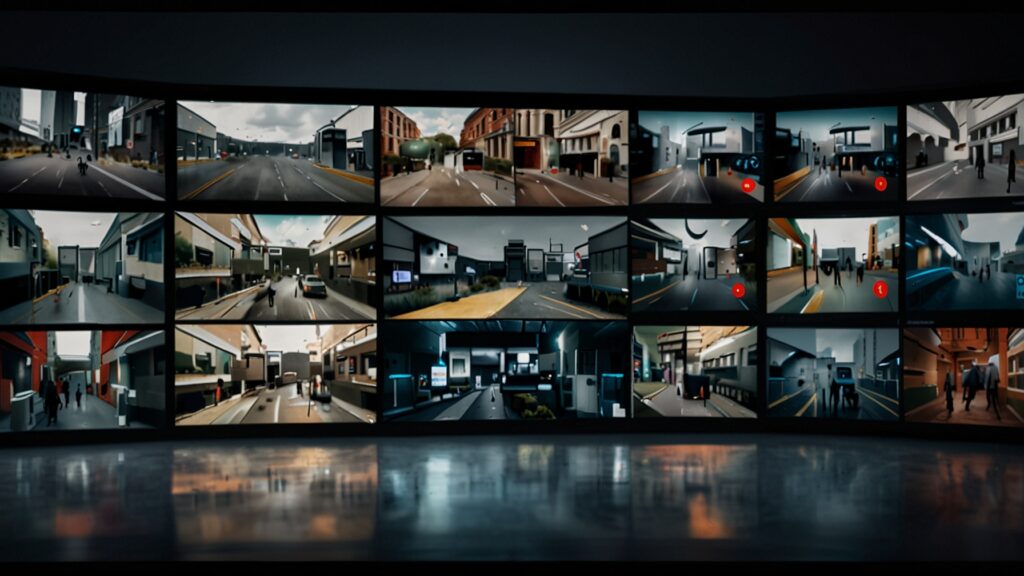
Security: Security firms utilize video analytics to realize real-time surveillance, thus ensuring facial recognition and behavior analysis to enhance security measures, preventing crimes and protecting assets.
Retail: Businesses use video analytics to track customer behavior for store layouts and customer service improvements, optimizing store operations and increasing sales.
Emerging Trends
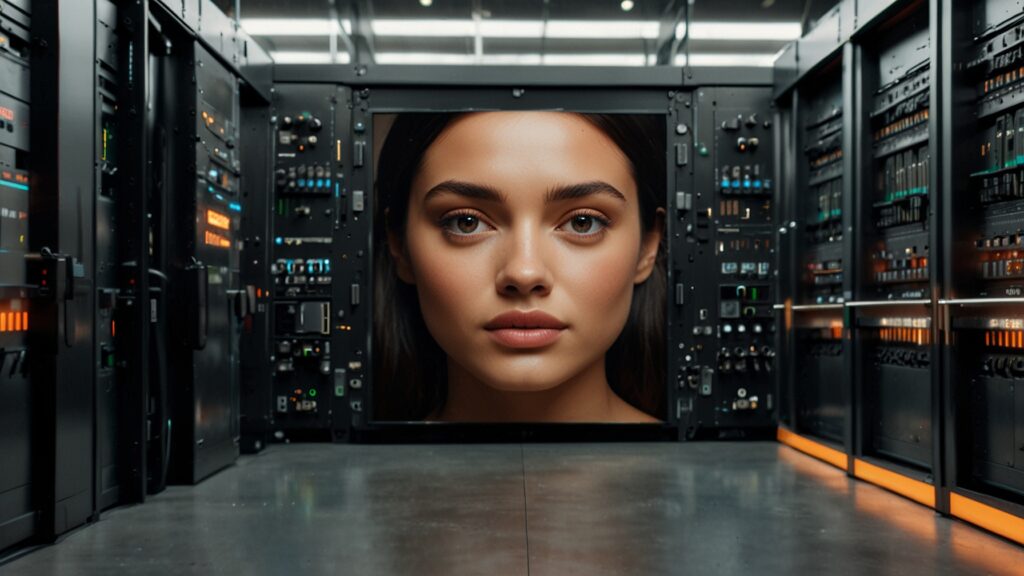
Real-time Processing: Advancements in real-time video processing are enabling more real-time insights, critical to many applications, ranging from autonomous driving to live event monitoring, driving innovation and efficiency.
Video Synthesis and Manipulation Detection: Tools for detecting deep-fakes and other instances of video tampering are becoming ever more vital, safeguarding trust and protecting against misinformation.
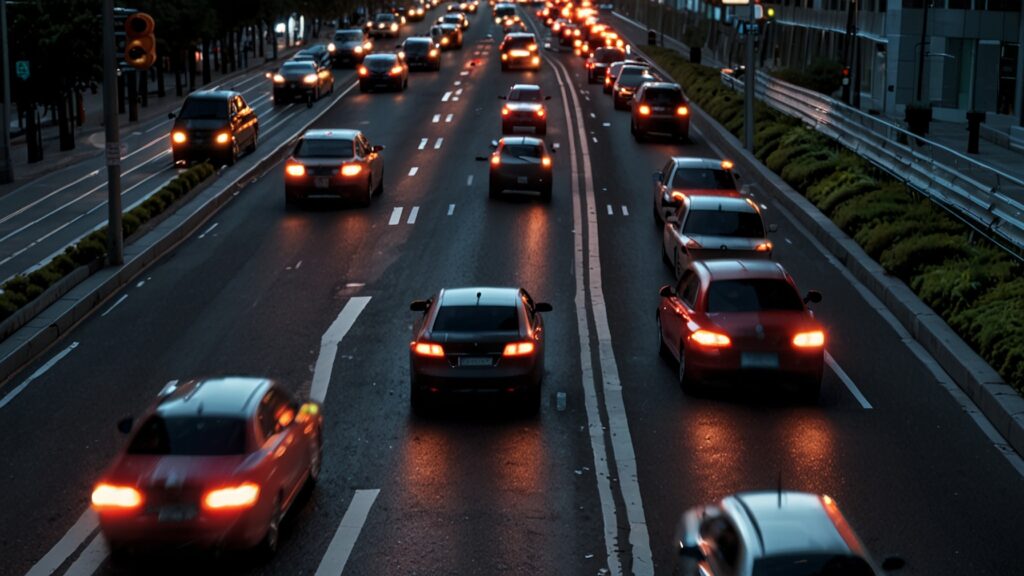
AI-driven Video Understanding: Extracting deeper meaning out of video using AI, including understanding of complex scenes, actions, and interactions, opening new possibilities for video search, content creation, and analysis.
Video Analytics for Smart Cities: Applications in urban planning, traffic management, public safety, and environment monitoring, contributing to sustainable and efficient urban development.
Privacy-preserving Analytics: New techniques being developed for the analysis of video data in such a way that privacy is preserved alleviate concerns about surveillance and data protection.
Conversational Analytics
It has oriented conversational analytics to understand customer sentiment and agent performance within interactions of humans, mostly relating to customer service or sales.
Examples and Case Studies
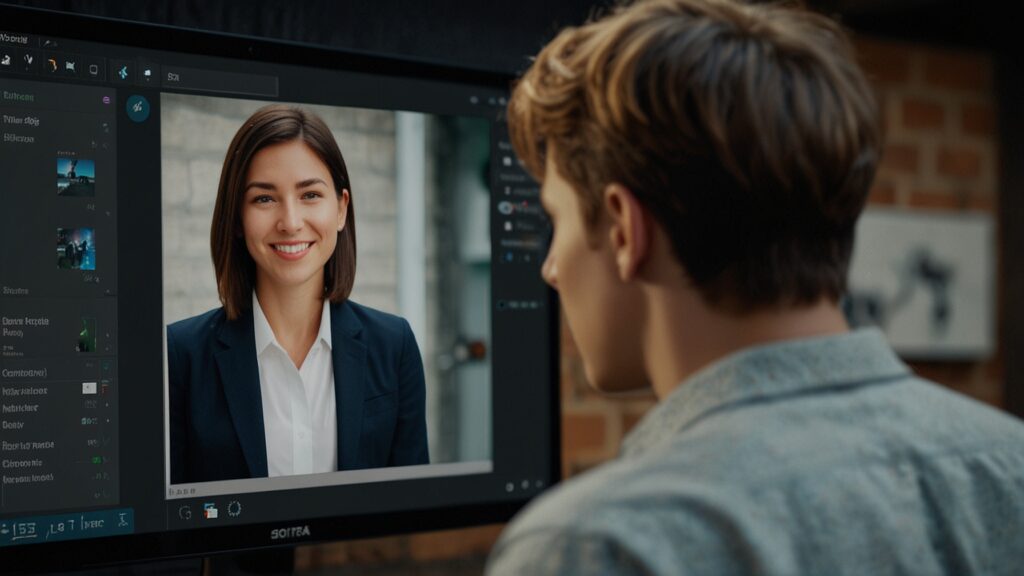
Customer Support: This is a methodology used by companies to analyze chat interactions for process improvement opportunities in agent performance or customer satisfaction, leading to higher customer satisfaction and loyalty.
Sales: Conversational analytics is applied to a sales call to see how the practices can be improved with conversational insights, resulting in increased sales and revenue.
Emerging Trends
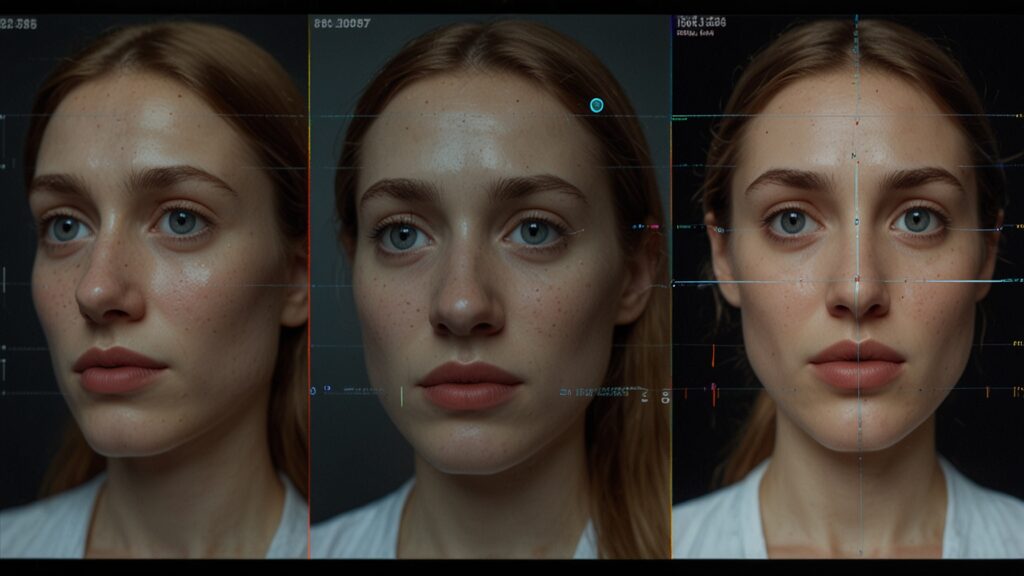
AI-Enhanced Dialogs: Generative AI, as used in conversational analytics, drives the evolution of more human-like and situationally aware virtual assistants and chatbots.
Emotion Detection: Advanced emotion detection in conversations is improving the ability to understand and react to customer needs better than ever.
Conversational AI for CX: Enhance customer experiences through highly personalized interactions and proactive support.
The Evolution of Analytics
The foundations for analytics can be further traced to the development of statistical analyses and data mining techniques in the early years of the 20th century. The process was accelerated with the introduction of computers, followed by the development of data warehouses and business intelligence tools.
Early Developments
Statistical Analysis: Analytics in the early days focused on the statistical methods that could interpret data trends and relationships.
Data Mining: Techniques that could discover patterns and relationships from large datasets emerged in the 1980s and 1990s.
Rise of Machine Learning and AI
Machine Learning: In the 2000s, there had been a proliferation of machine learning algorithms that substantially increased accuracy and efficiency for data analysis.
Deep Learning: Deep learning, especially neural networks, had been instrumental in having breakthroughs related to image and speech recognition.
NLP and Augmented Analytics
Natural Language Processing: These techniques transformed text and speech analytics to have a much more depth-of-knowledge grasp of the human language.
Augmented Analytics: The evolution of augmented analytics recently occurs with the backing of AI to make the analysis and the discovery of insights much more intuitive
Use Cases Across Industries
Customer Service
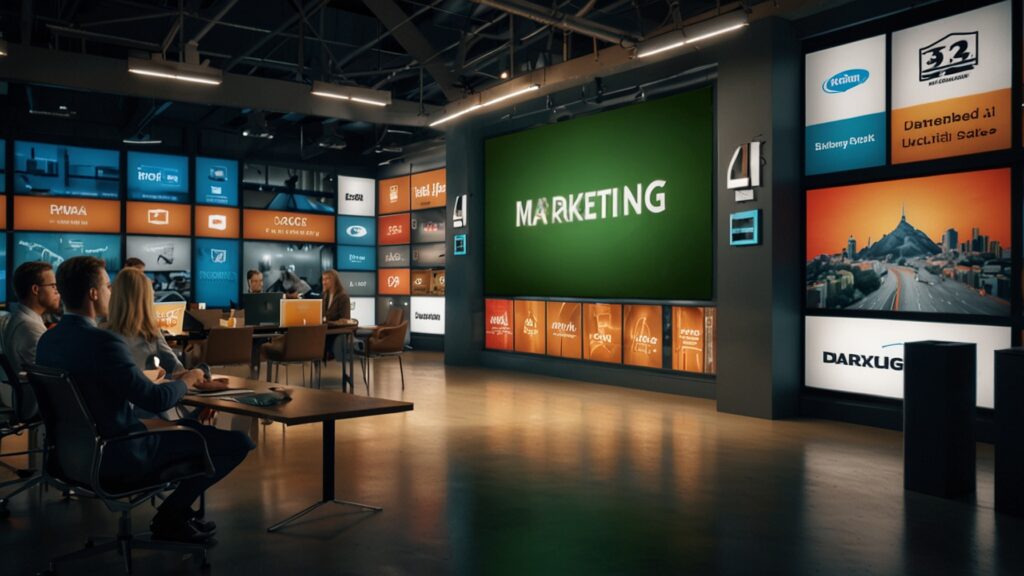
Speech Analytics: Companies are using speech analytics during customer conversations for quality purposes and agent training.
Marketing Text Analytics: Social media companies run text analytics on their platforms to monitor brand sentiment and optimize their marketing campaigns.
Video Analytics: Ad tech companies use video analytics in their targeted advertising and audience measurement.
Conversational Analytics: Chatbot conversation is analyzed to optimize the customer’s experience and satisfaction.
Healthcare
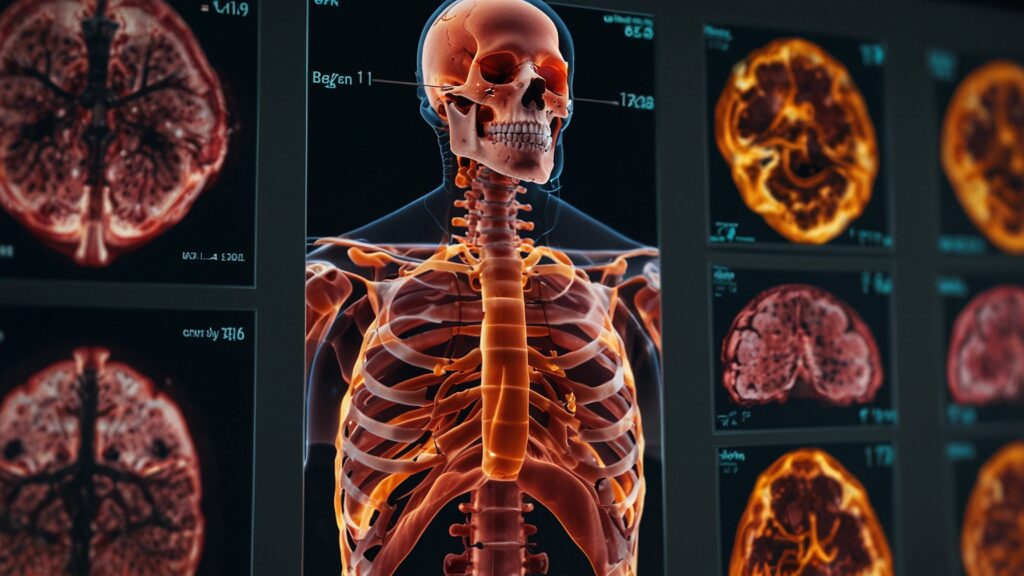
Voice and Audio Analytics: Healthcare providers use voice analytics in monitoring and diagnosis, while audio analytics is useful in the maintenance of medical equipment, improving patient outcomes and operational efficiency.
Video Analytics: Video analytics is done in medical imaging technologies to enhance the accuracy of the diagnostics, aiding in early disease detection and treatment planning.
Security
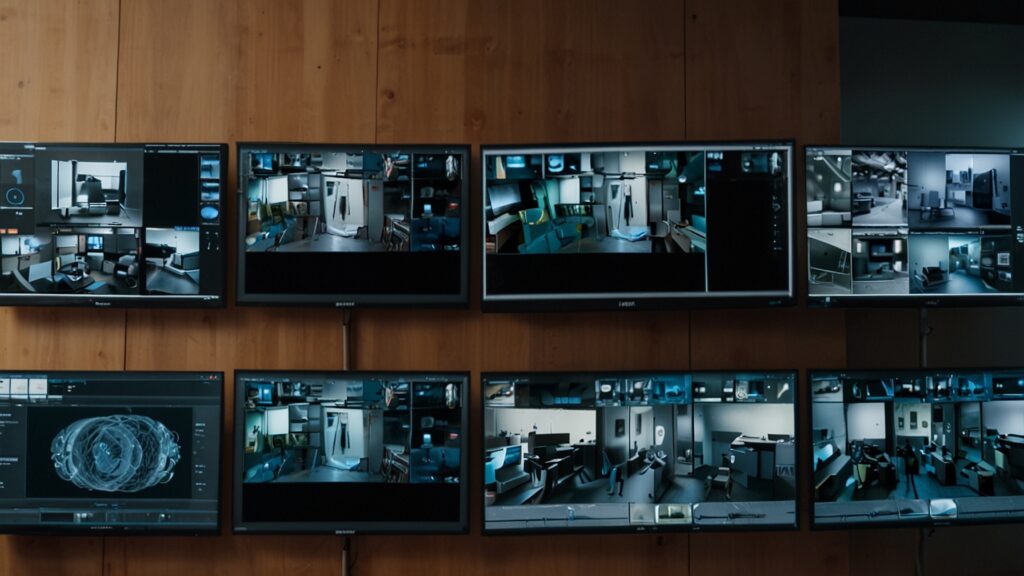
Video Analytics: Surveillance systems use video analytics to identify threats in real–time and identify faces, enabling rapid response to incidents and improving overall security.
Audio Analytics: Audio analytics measures the noise levels in the security environment and detects anomalies, such as glass breaking or gunshots, providing early warning of potential threats.
Tunk.ai’s SpIn: A Game-Changer with Anya
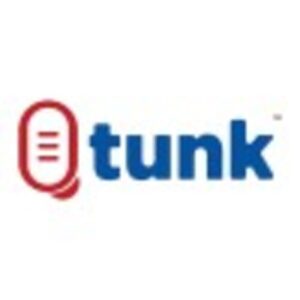
Tunk.ai is revolutionizing how businesses extract value from spoken language. SpIn changes raw audio into actionable insights, making it possible for companies to truly understand their customers.
At the heart of this powerful tool is Anya, Tunk.ai’s intelligent chatbot. Anya is an essential partner in supercharging SpIn’s capabilities. Equipped with the latest natural language processing and machine learning capabilities, Anya can summarize long conversations in a second, detect key points, and extract sentiments.
This means that businesses can now get into the weeds of their customers’ interactions to turn up hidden trends and preferences at speeds and accuracies unimaginable earlier.
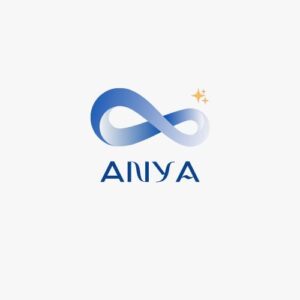
For example, with SpIn powered by Anya, Tunk.ai can analyze customer interactions in just minutes, identifying common issues and areas of improvement. Or, it could evaluate sales calls for effective sales pitches to deliver the most when it comes to sales strategies. The possibilities are endless.
Anya can transform lengthy earnings calls into concise summaries within seconds. Anya not only extracts key talking points but also dives deeper, pinpointing sentiment and uncovering crucial financial insights that might otherwise be buried within the conversation. Integrating SpIn’s robust data analysis with Anya’s intelligent insights, Tunk.ai enable businesses a one-stop solution to unlock the full potential of their spoken data.
The Future of Interaction Analytics
The future of analytics is increasing sophistication, integration, and real-time capabilities. Some of the key trends include.
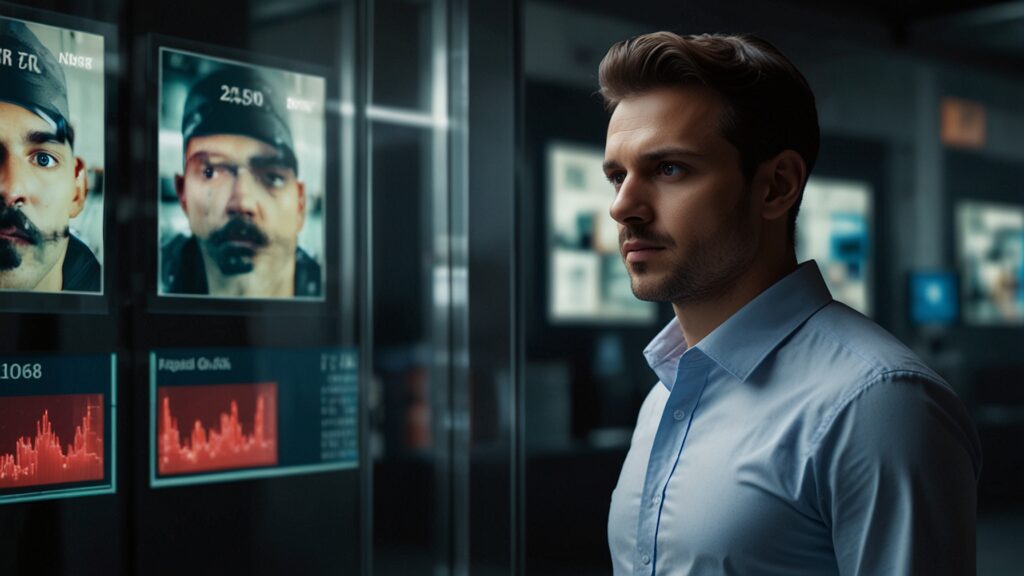
AI-driven insights: Advanced AI algorithms are making it possible to go deep into complex patterns of data and come up with more accurate predictions and recommendations. AI-driven insights will feature more and more as an integral part of decision-making processes across industries.
Real-Time Analytics: The processing and analysis of data in real-time is increasingly becoming very essential in areas like fraud detection, customer service, and autonomous systems.
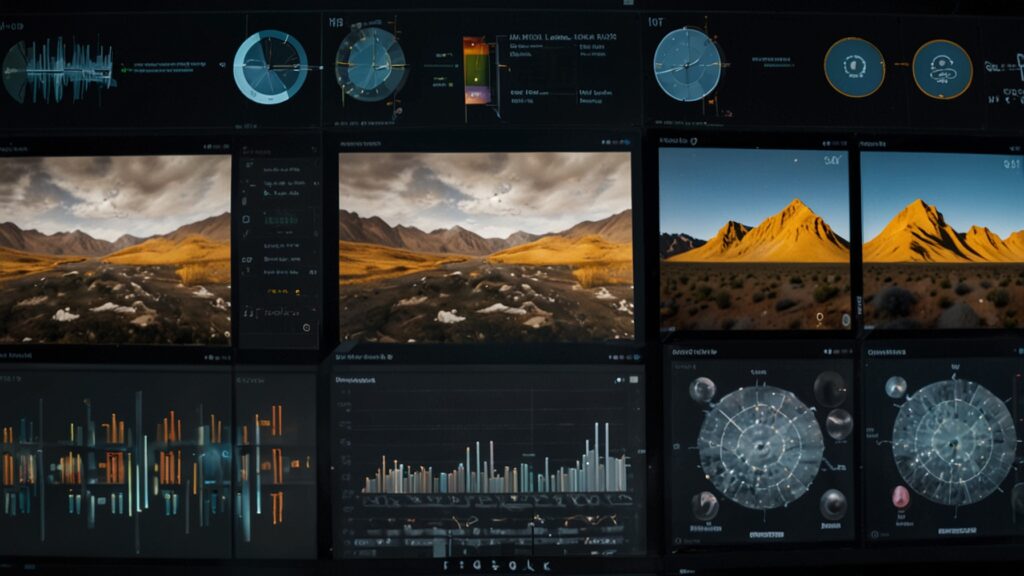
Multimodal Analytics: Incorporating textual, audio, and video data as sources will help gain holistic understanding about complex phenomena. Multimodal analytics allows insight into these dimensions and develops holistic solutions.
Explainable AI or XAI helps build trust with increased interpretability of AI models, empowering better decisions. Explainable AI is going to make AI transparent and let users understand and validate the insights that it drove.
With the proliferation of analytics in increasing pervasiveness, one of the very important aspects will be to address ethical concerns regarding data privacy, bias, and transparency. The need will arise to create ethical guidelines and frameworks to ensure trust and accountability.
Conclusion
Analytics has traveled a long journey from being a statistical tool to a strategic asset with the power to transform businesses and society. Many valuable insights can be unlocked by organizations, many operational dysfunctions can be smoothened, and they can seek competitive advantage by simply understanding the nuances of voice, audio, speech, text, video, and conversation analytics.
Other emerging trends—in areas like augmented analytics and generative AI—further open the realization possibilities for analytics in driving innovation to solve complex challenges. Continued advances in technology will keep these different analytics domains together in evermore sophisticated and intuitive systems, redefining how we work with data and with each other.
The future in the field of analytics is promising, with quite a number of areas bound to show much progress and improvement in the quality of life.